Process mining offers a data-driven approach to process discovery by analyzing event logs from enterprise software systems. This can be a game-changer, providing a more objective view of processes compared to traditional methods that rely on manual interviews or anecdotes. However, process mining also has limitations. Data quality issues in event logs can hinder analysis, and integration with various software systems can require upfront development effort.
Welcome to part 2 of an ongoing series of blog posts demystifying "process intelligence" - the terms, the technologies, and how and why process intelligence can help improve your business. Following part 1 which focused on manual process discovery (and why it doesn't work), this post will discuss a common technology based approach to process discovery, called process mining. As always, you can learn more about this and related topics by reading the Skan Process Intelligence Playbook here.
The term Process Mining became broadly popularized in 2011 with the release of the “The Process Mining Manifesto”, published by the “IEEE Task Force on Process Mining”, led by Dr. Wil van der Aalst (known as the “Godfather of Process Mining”)
The Process Mining Manifesto defines process mining as follows:
“Process mining techniques are able to extract knowledge from event logs commonly available in today’s information systems. These techniques provide new means to discover, monitor, and improve processes in a variety of application domains.”
So what does this actually mean? How exactly can process mining help with process optimization? And equally important, where does it fall short in achieving these goals?
According to the Gartner 2020 Process Mining Market Guide, “The starting point for any process mining task is an event log."
An event log is a timestamp produced by a piece of software. These event logs are essentially database entries that signify a specific action took place in the software at a specific time. Simple examples of one of these actions is the creation of a new customer account, or updating customer account information in a software system, such as SAP, Oracle, or other line of business applications such as FienOS. (software commonly used in the insurance industry)
Process Mining tools are able to extract these event logs by integrating directly to these enterprise software systems. Then, by applying statistical analysis to all of the event logs across these different systems, process mining is able to build a data-driven view of how a process is being completed.
This approach was a paradigm shift towards understanding how work was being done. Rather than relying on interviews or anecdotal feedback, process mining tools use definitive data from the collected logs. By applying analytics tools and models on top of those data points, process mining can build accurate, data-driven views of how a process has historically been completed.
Despite this game changing shift process mining has enabled, there have also been several challenges that have emerged with these event log-based process mining techniques.
Challenges with event log based process mining
• Data quality issues: Similar to any other database, event logs often have data quality issues such as incorrect timestamps, noisy data and missing data attributes. Correcting these challenges often requires significant up front effort and support from IT teams to clean up data before being able to start the actual process analysis. According to the 2020 Gartner Process Mining Market Guide, "Typically, 80% of the effort and time are spent on locating, selecting, extracting and transforming the process.... Process mining often reveals data quality problems that need to be dealt with urgently”.
• Integration effort: Because process mining works by analyzing software event logs, it needs to be integrated with the software, which requires up front development effort and support from IT teams. This can slow down and complicate the rollout of process mining projects.
• Many process steps are not visible from an event-log: Process Mining can only identify process steps in software that have event logs. However, many pieces of software do not provide software logs. Additionally, even within software that does provide logs, those logs do not provide visibility into all possible actions. As a result, while process mining can provide a great high level view of a process, it can often misses significant steps and details in a process.
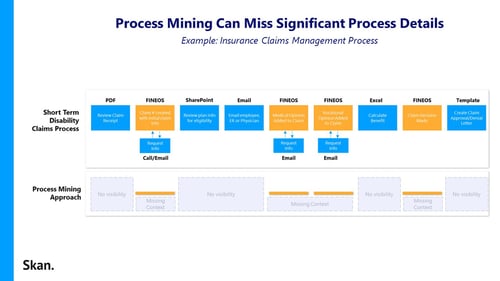
• The paradox of process mining - 'you need process knowledge to get process knowledge': Successful implementation of process mining often requires existing knowledge of a process, in order to guide the necessary integration. In fact, according to Deloitte's 2021 Global Process Mining Survey, the most 'critical skills' for the success of a process mining implementation was "Process Knowledge". It is counterintuitive that the most critical success factors for getting value from a technology that is supposed to deliver process visibility is existing process visibility.
Ready to learn more? Read the Process Intelligence Playbook
Modern process optimization requires a data-driven approach.That means considering all the technologies that can help you do that.
But, where do you start?
Skan’s actionable Process Intelligence Playbook explains approaches to process discovery, how to get started with process intelligence, and illustrates process intelligence in action through real-world applications.
Ready to learn more? Access the Skan Process Intelligence Playbook today.